brain of mat kelcey...
keras3 to jax to tflite/liteRT via orbax
November 25, 2024 at 02:10 PM | categories: tflite, litert, keras3, jax, orbax
vmapped keras3 model inference in tflite
yolz; you only look o̶n̶c̶e̶ zero times
October 26, 2024 at 06:45 PM | categories: keras3, jax
zero shot object detection in kera3 jax
differentiable kalman filters in jax
March 29, 2024 at 06:45 PM | categories: jax
kalman filters have numerous parameters to tune, why not roll them out with jax and use backprop to fit them
a (larger) wavenet neural net running on an FPGA at (almost) 200,000 inferences / sec
October 12, 2023 at 01:00 PM | categories: eurorack, wavenet, fpga
wavenet on an mcu is fast, but, oh boy! wait until you see an even larger model running on an FPGA!
a wavenet neural net running on a microcontroller at (almost) 50,000 inferences / sec
September 09, 2023 at 09:00 PM | categories: mcu, wavenet, eurorack
wavenet can run at audio rates on an mcu, if you cache carefully, and be used for fun eurorack effects.
high performance ML with JAX
September 12, 2021 at 12:30 PM | categories: jax, talk
did a talk ay pycon on jax. check out the recording!
evolved channel selection
March 01, 2021 at 10:20 PM | categories: projects, ga, jax
rather than use all 13 channels in a multi spectral image for classification can we train a model that is robust to all combos, at all resolutions, and use a genetic algorithm to choose which are the most valuable? (spoiler; yes)
crazy large batch sizes
February 14, 2021 at 10:30 PM | categories: quick_hack, tpu, jax
a quick hack to see how fast we can get a v3-32 pod slice cranking with a global batch size of 170,000; tl-dr pretty fast!
solving y=mx+b... with jax on a tpu pod slice
February 07, 2021 at 01:00 PM | categories: tpu, ensemble_nets, jax, projects, haiku
a 4 (and a bit) part tutorial / colab / screencast series starting with jax fundamentals working up a data parallel approach to running on a cloud tpu pod slice... all focused on solving the toughest problem in machine learning; 1d y=mx+b
develomentor.com podcast interview
December 07, 2020 at 05:00 PM | categories: talk
was a guest on the develomentor podcast talking about random parts of my career
out of distribution detection using focal loss
December 02, 2020 at 01:00 PM | categories: objax, jax, projects
a series of small experiments on using focal loss to do out of distribution detection
my updated list of cool machine learning books
November 01, 2020 at 09:40 PM | categories: Uncategorized
it's been ten years so it's probably time to update my list of cool machine learning books.
dithernet very slow movie player
October 21, 2020 at 10:30 PM | categories: gan, jax, projects, objax
a GAN experiment to generate dithers for an eink screen minimising pixel change between frames for a very slow movie player.
ensemble networks
September 17, 2020 at 06:30 AM | categories: objax, projects, ensemble_nets, jax
ensemble nets; using jax vmap to batch over not just the inputs of a model but also sets of multiple models parameters.
metric learning for image similarity search in objax
September 02, 2020 at 12:00 PM | categories: objax, metric_learning, jax
an objax tutorial on using contrastive learning for image similarity.
objax on honeysuckle farm
August 30, 2020 at 02:45 PM | categories: talk
i think high level short explainer videos on jax frameworks while doing farm chores is going to be a growing genre.
the map interpretation of attention
August 19, 2020 at 10:30 AM | categories: talk, three_strikes_rule
a talk i did at melbourne ml/ai on how attention mechanism can be interpretated as a form of differentiable map. check out the recording!
self supervised learning and making use of unlabelled data
July 02, 2020 at 05:00 PM | categories: talk
a recording of a talk i did on self supervised learning at yow data.
a jax random embedding ensemble network
June 15, 2020 at 06:30 AM | categories: ensemble_nets, jax
random embedding networks can be used to generate weakly labelled data for contrastive learning and can be run in single model ensembles as a single forward pass in jax.
keras.io post on metric learning for image similarity search
June 05, 2020 at 12:00 PM | categories: metric_learning, keras
a keras.io tutorial on using contrastive learning for image similarity.
an illustrative einsum example
May 27, 2020 at 12:00 AM | categories: talk, short_tute
code (and youtube walkthrough) of a port of some numpy code i did recently to einsum that i thought was illustrative.
using cross entropy for metric learning
May 19, 2020 at 06:00 PM | categories: metric_learning, talk
youtube link and slides of a talk i did recently on contrastive learning at the melbourne ml and ai meetup
measuring baseline random performance for an N way classifier
April 11, 2020 at 12:34 PM | categories: short_tute, three_strikes_rule
quick example code of a simple way to gauge baseline random performance.
deriving class_weights from validation data
March 03, 2020 at 06:00 PM | categories: short_tute, three_strikes_rule
quick example code demoing a way to derive class_weights from performance on validation data. this can often speed training up.
initing the biases in a classifer to closer match training data
February 27, 2020 at 12:00 PM | categories: short_tute, three_strikes_rule
some code showing how you can init the bias of classifier to match the base distribution of your training data.
minimal example of running pybullet under google cloud dataflow
January 29, 2020 at 12:00 AM | categories: short_tute
some code that demos how to run pybullet for generating a truck load of synthetic training under google cloud dataflow.
data engineering concerns for machine learning products
September 26, 2019 at 06:00 PM | categories: talk
slides of a talk i did at the melbourne data engineering meetup.
solving cartpole... by evolving the raw bytes of a 1.4KB tflite microcontroller serialised model
September 13, 2019 at 12:00 AM | categories: projects
evolving a controller for cartpole using an evolutionary algorithm that operates directly on the byte level of a serialised tf lite microcontroller model.
brutally short introduction to learning to learn
August 07, 2019 at 12:00 AM | categories: talk
recording of a talk i did on meta learning at yow data
a half baked pix2pix experiment for road trip videos with teaching forcing
June 26, 2019 at 01:00 PM | categories: gan, projects
a half baked attempt to train a pix2pix model on dash cam videos from a roadtrip around the eastern state of the u.s.
pybullet grasping with time contrastive network embeddings
June 11, 2019 at 01:00 PM | categories: projects
an example of using time contrastive networks to learn embeddings for the pose of a kuka arm in a pybullet simulated grasping environment.
natural questions a benchmark for question answering research
February 01, 2019 at 12:00 AM | categories: paper
the last paper i was involved in at google has been released! congrats to tom and the team.
counting bees on a rasp pi with a conv net
May 17, 2018 at 12:30 PM | categories: projects
training a fully convolutional unet to count bees from a raspberry pi stuck to the side of a hive.
fully convolutional networks
April 06, 2018 at 06:00 PM | categories: short_tute
a short walkthrough explainer on fully convolutional networks.
using simulation and domain adaptation to improve efficiency of deep robotic grasping
September 22, 2017 at 12:00 AM | categories: paper
a paper i helped with at google robotics has been released! congrats to konstantinos and the team!
deep reinforcement learning for robotics
September 21, 2017 at 07:12 PM | categories: talk
a recording of a talk i did at the melbourne ml ai meetup.
simple tensorboard visualisation for gradient norms
June 27, 2017 at 09:45 PM | categories: Uncategorized
some cook book examples of gradient norm visualisation in tensorboard.
after 2,350 days in america we are moving home...
June 14, 2017 at 10:00 PM | categories: Uncategorized
i'm leaving google brain and we're moving back to australia.
cartpole++
August 11, 2016 at 10:00 PM | categories: projects
a pybullet 3d version of cartpole where the pole isn't connected to the cart and you have to learn from pixels.
wikireading a novel large-scale language understanding task over wikipedia
August 11, 2016 at 12:00 AM | categories: paper
our wikireading paper is out!congrats to daniel and the team!check it out at on arxiv...WikiReading: A Novel Large-scale Language Understanding Task over Wikipedia...
learning to do laps with reinforcement learning and neural nets
February 13, 2016 at 10:00 PM | categories: projects
using reinforcement learning to train neural nets for driving a simulated robot around a track.
brutally short intro to theano word embeddings
March 28, 2015 at 01:00 PM | categories: Uncategorized
one thing in theano i couldn't immediately find examples for was a simple embedding lookup table, a critical component for anything with NLP. turns out that it's just one of those things that's so simple no one bothered writing it...
hallucinating softmaxs
March 15, 2015 at 10:00 PM | categories: Uncategorized
language modelling is a classic problem in NLP; given a sequence of words such as "my cat likes to ..." what's the next word? this problem is related to all sorts of things, everything from autocomplete to speech to text.the...
theano and the curse of GpuFromHost
February 22, 2015 at 10:00 PM | categories: Uncategorized
i've been reviving some old theano code recently and in case you haven't seen it theano is a pretty awesome python library that reads a lot like numpy but provides two particularly interesting features.symbolic differentiation; not something i'll talk about...
dead simple pymc
December 27, 2012 at 09:00 PM | categories: Uncategorized
PyMCis a python library for working withbayesian statistical models,primarily usingMCMCmethods. as a software engineer who has only just scratched the surface of statistics this whole MCMCbusiness is blowing my mind so i've got to share some examples.let's start with the...
smoothing low support cases using confidence intervals
December 08, 2012 at 10:50 PM | categories: Uncategorized
say you have three items; item1, item2 and item3 and you've somehow associated a count for each against one of five labels; A, B, C, D, E> data A ...
item similarity by bipartite graph dispersion
August 20, 2012 at 08:00 PM | categories: Uncategorized
the basis of most recommendation systems is the ability to rate similarity between items. there are lots of different ways to do this. one model is based the idea of an interest graph where the nodes of the graph are...
finding names in common crawl
August 18, 2012 at 08:00 PM | categories: Uncategorized
the central offering from common crawl is the raw bytes they've downloaded and, though this is useful for some people, a lot of us just wantthe visible text of web pages. luckily they've done this extraction as a part of...
fuzzy jaccard
July 31, 2012 at 08:00 PM | categories: Uncategorized
the jaccard coefficient is one of the fundamental measures for doing set similarity. ( recall jaccard(set1, set2) = |intersection| / |union|. when set1 == set2 this evaluates to 1.0 and when set1 and set2 have no intersection it evaluates to...
Next Page »
popular posts...
FPGA wavenets : eurorack audio processing neural nets running at ~200,000 inferences/sec (oct 2023)
dithernet very slow movie player : a GAN that slowly plays a movie over a year on an eink screen (oct 2020)
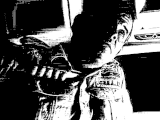
evolved channel selection : neural networks robust to any subset of input channels, at any resolution (mar 2021)
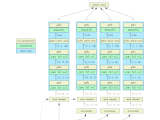
ensemble nets : training ensembles as a single model using jax on a tpu pod slice (sept 2020)
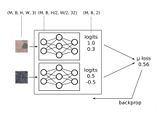
bnn : counting bees with a rasp pi (may 2018)
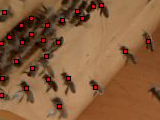
drivebot : learning to do laps with reinforcement learning and neural nets (feb 2016)
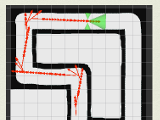
wikipedia philosophy : do all first links on wikipedia lead to philosophy? (aug 2011)
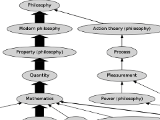
some papers from my time at google research / brain...
- Natural Questions: a Benchmark for Question Answering Research
- Using Simulation and Domain Adaptation to Improve Efficiency of Deep Robotic Grasping
- WikiReading: A Novel Large-scale Language Understanding Task over Wikipedia
my honours thesis
the co-evolution of cooperative behaviour (1997) evolving neural nets with genetic algorithms for communication problems.
old projects...
- latent semantic analysis via the singular value decomposition (for dummies)
- semi supervised naive bayes
- statistical synonyms
- round the world tweets
- decomposing social graphs on twitter
- do it yourself statistically improbable phrases
- should i burn it?
- the median of a trillion numbers
- deduping with resemblance metrics
- simple supervised learning / should i read it?
- audioscrobbler experiments
- chaoscope experiment